psyPhon: Use Case
What’s Happening? You don’t seem your normal self? using psyPhon to find outlier behavior from text.
We’ve all had the experience of noticing a friend, colleague or co-worker on a day they are a bit off their game. Could be a they are a bit more stressed than usual, or unusually chirpy! Then we talk to them and find out what’s been bugging them or what’s got them that bounce in their step. There are three parts to this:
- We’ve known the person for a bit. In other words we have a baseline range for their behavior
- We notice that they are above or below their baseline range
- We engage with them to figure out what’s going on.
With psyPhon you can do the same with any corpus of text generated by a person or conceptually cohesive group.
Any corpus?
Any person?
Any conceptually cohesive group?
What does that mean?
Ok, let’s start from the bottoms up. Any “conceptually cohesive group” … Let’s look at some examples and the prudent ‘grain or fistfuls of salt’ we should take with the conclusions. Let’s say we take all the English language tweets worldwide and label it as a “conceptually cohesive group” is that valid? The answer is a resounding “maaaybee” \_(ツ)_/¯ Because it depends what is that going to be used for. If this “conceptually cohesive group” is going to be used to linguistically identify potential terrorist threats, the answer is a clear “N-O… that’s not a valid grouping! there is too much variability in the group for such a serious decision”… on the other hand if the data will be used to bubbler up topics for a team to include in an online trivia game, the answer is “Sure, you may start here”… if the grouping is “All yelp reviews of McDonalds” and it to be used to bubble up major issues by region.. then… “okay… but take it with a grain of salt and check with the reginal managers”.. if it’s “all the yelp reviews for the McDonalds at the corner of Lamar and Barton Springs” then any conclusions will carry more weight… If the group is “emails of all the employees of the IT department” and it’s to be used to compare stress level of the IT department vs the Customer Care department… then “okay some high level conclusion may be valid, again double check with the managers”… if the corpus is “all the emails over the last year for the CIO” and it’s to be used to identify her normal range of stress and find the content that threw her off… the answer is … “That’s a tight corpus, focused on one person… let’s see what we find”
If this does not make sense, click here for a complimentary consulting session. On the other hand, if it does make sense let’s look at a real world use case. A Texas insurance company had been solvent and in the black for over 5 years then within 6 weeks it imploded with $60 million in losses/ Leaving thousands of home, auto and business insurance policy holders in limbo. The State had to step in to cover them and assigned the investigation of ‘what happened’ to a reputable Texas law firm which used psyPhon to see if and how they could build an case of about $60 million in damages against the former owners and managers for fraud.
psyPhon processed the text of thousands of emails written by the nemployees and executives in the 19 months leading up to the collapse . Using algorithms based on cognitive linguistics [1] it assigned a numerical value between 0 and 1 to the level of stress or internal conflict in each email, represented by the difference in the two types of thinking- unconscious and rational [2]. The level of stress was plotted over time for the CEO.
The following illustrates how psyPhon:
- Established a baseline live of stress of the CEO
- Filtered out outliers
- Got an idea of what was going on.
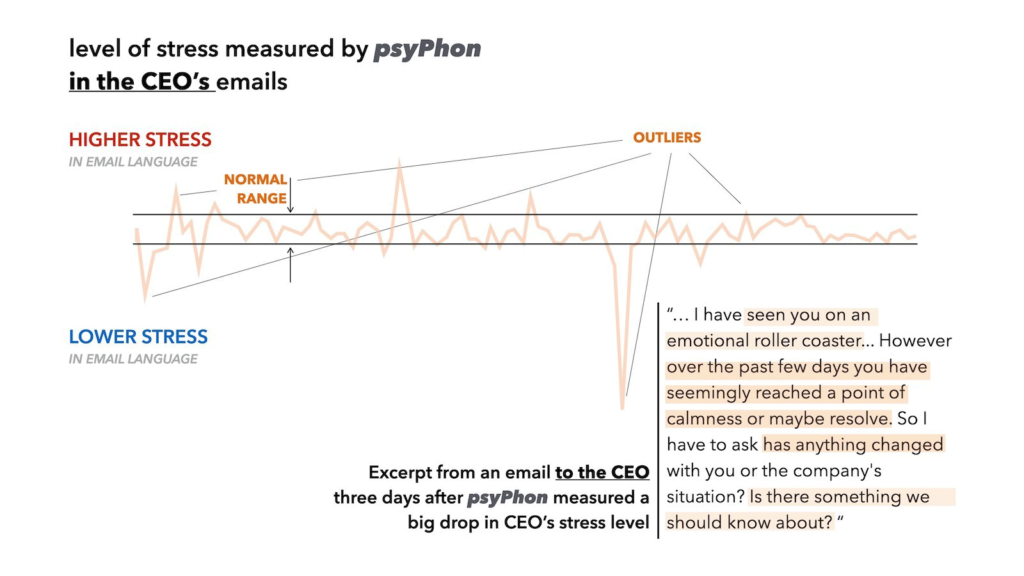
Since this is not a case study, only one peculiar point is highlighted here. The case study of the actual investigation is in a separate publication.
This use of psyPhon enables any platform that is collecting text, to pick a psychological or emotional measure like ‘stress’ or ‘internal conflict’; Plot it over time and dial in what would be considered a ‘normal range’ of stress or variation of that measure; Tag the outliers and then study them. In the above example the CEO’s level of ‘stress’ or ‘internal conflict’ took a huge drop at one point. Emails from her CFO a few days later show that her staff noticed the change, and expressed concern by stating that she seems to have reached a point of calmness or maybe resolve. The unfamiliar behavior made the CEO inquire about the cause. Though anecdotal in nature, the example is an interesting validation of psyPhon’s ability to detect such shifts in text as may be picked up by people in person.
[1] “Cognitive Linguistics is a modern school of linguistics which Investigates the relationship between, Language, Thinking and Socio-physical experience” Cognitive Linguistics: A Complete Guide, Vyvyan Evans, Edinburgh University Press, 2019
[2] “Thinking, Fast and Slow, Daniel Kahneman, Farrar, Straus and Giroux, 2011” Presents a model of cognition and thinking as two types. Type 1 thinking operates automatically and quickly, with little or no effort and no sense of voluntary control (unconscious). Type 2 thinking allocates attention to the effortful mental activities that demand it, including complex computations (rational)